Beyond the biological contagion – pathogens’ jump from one person to the next and between locations -, there is another element of contagion, of a more social nature, that may also affect infection processes. This is the spread of ideas and thoughts (i.e. norms around health behaviors like alcohol and smoke consumption, prevention measures adoption, treatments adherence). Individuals’ behavior factors have become key drivers for disease transmissions, at the centre of disease dynamics and control investigations.
Traditional surveillance capacity, which relies on reporting and confirmation of cases seen in health facilities, suffers from poor and at times slow information flows. In low- and middle-income countries, in particular, the parts of the world that are often most vulnerable to emerging epidemic-prone infectious disease threats, limited access to healthcare facilities as well as inadequate information infrastructures make it tough to meet surveillance needs. However, valuable health information is already being exchanged via social network, news outlets and mobile devices and appears to be a bold promise to assist traditional global public health efforts, as an additional information layer [1-3].
A large fraction of what we do, think and say is becoming digital, thus accessible and amenable to analysis. Data about human behavior and social interactions are nowadays produced and made available at an unprecedented pace. They are recorded at very high frequency, and are massive in size because of the explosion of mobile phone and Internet usage.
Mobile phones have become one the main sensors for users’ habits, even in many developing countries, where the penetration rates range from 65% in Uganda to 83% in Ghana [4]. In 2014, the United Nations called for an effort to use data and mobile phone data in support of the Sustainable Development Goals [5].
The call detail records (CDRs), automatically generated by our phones and recorded at large-scale by carriers for billing purposes, contain finegrained information on who called whom for how long and from where. Consequently, they have the potential to fundamentally transform the way we fight diseases.
We here report on a framework to extract insightful behavioral information from these rich data streams and on how they can be used to augment our understanding of a disease contagion dynamics, then to predict its course, and finally to develop ways of controlling it.
EPIDEMIC MODELING: SOCIAL THINKING
When we think about infectious diseases spreading, we have to think about individuals, who wander around across several locations, from time to time are in direct or indirect contact with others, and transmit infectious pathogens or disease vectors (e.g. mosquitos, ticks) they carry with them.
Traditional epidemics modeling approaches have been based on the assumption that such mobility and contact events occur at random. However the dynamics cannot be so simple: there is a structure of the society that spatially organizes individuals, in recurrent and heterogeneous pattern of contacts.
Modern epidemic modeling recognizes the importance of population structure and patterns of interactions and mobility, as elements that can substantially alter the likelihood of disease propagation. The first step toward the consideration of this complexity is the integration of location information into traditional epidemic model. What routes do people travel through? Who do they interact with? These questions may now be answered thanks to the proliferation of mobile phones.
In this short article (see [6] for a full version), we present an epidemic model to describe a hypothetical countrywide-scale infectious disease spread, based real-world dataset of CDRs from Ivory Coast. A natural population living in any considerable geographic area (e.g., a country) will be composed of a number n of local populations (i.e., sub-populations), occupying n corresponding sub-areas, which interact and exchange individuals between them, because of their movement, through a given network of mobility inferred from mobile phone traces. Within each geographic subarea, sub-populations may be in contact and may change their health state according to the disease dynamics. By doing so, the system will evolve under the action of two processes, namely the disease contagion and the mobility of individuals.
As a first step we model the process of disease spread using a SEIR epidemiological model. It essentially models the evolution of a disease as individuals’ transitions among different health states (here SEIR, i.e. Susceptible, Exposed, Infected and Recovered). The extent of these transitions depends on parameters that are disease-specific. We used parameters values estimated from the Ebola outbreak in Sierra Leone in 2014.
Simultaneously with the contagion process, individuals move among the geographic sub-areas.
So, as a second step of our work, we quantified these movements using the CDRs data. As a matter of fact, every time a person uses a phone, makes a call, sends an SMS or goes online, a CDR is generated. The record contains the caller and callee IDs, timestamp, duration and type of communication, as well as an identifier of the cellular tower that handled the call.
Individuals’ daily locations and movements can be then aggregated to quantify the mobility among 508 Ivorian administrative regions called subprefectures. Overall, the final model is given by the combination of these two layers and can be described graphically by figure 2.
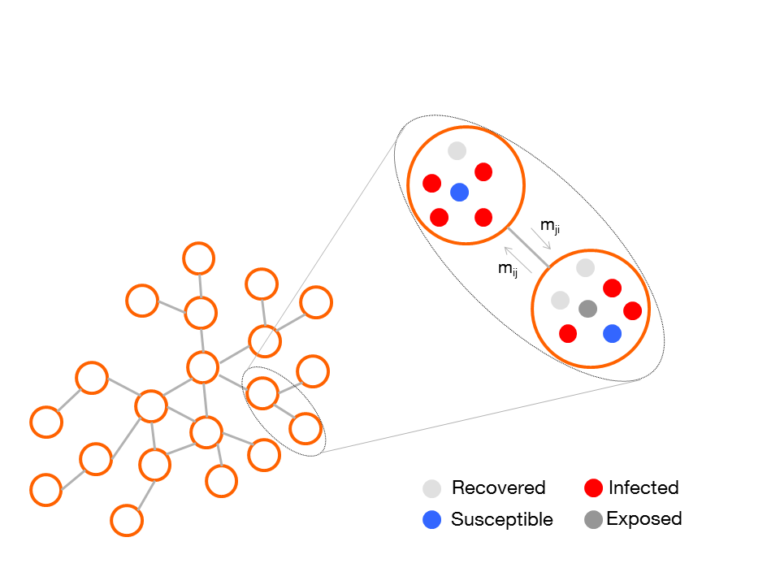
Figure 2 – Schematic illustration of the epidemiological model with SEIR dynamics.
On the left side, the sub-populations network consists of patches connected among them according to individuals’ moving. In each sub-population, the disease transmission occurs according to the SEIR model. Then individuals move among patches according to a specific mobility pattern described by transition probabilities (i.e., mij and mji).
We will be then able to study the epidemic evolution over the time to eventually forecast its course and so communicate its severity if left unchecked (figure 3).
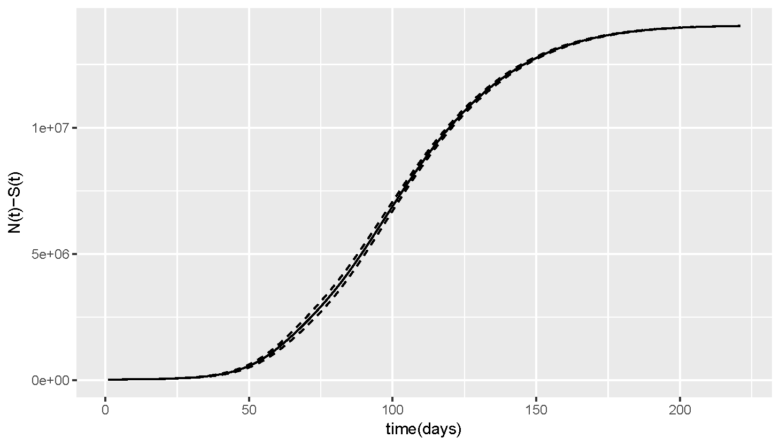
Figure 3 Total number of infections since the beginning of the simulations, over a seven-month period
TOOLS FOR PUBLIC ACTION
Beyond the simple forecast, models are extremely relevant to assess the effect of containment efforts and provide guidance on the scale of interventions. Disease spread is, in fact, a highly heterogeneous process, with certain areas (or indeed individuals) being at higher-risk than others. Understanding such an heterogeneity is crucial for hindering disease spread while optimising the use of available resources.
It has been shown that systematic variations in mobility patterns of the population are sufficient to drive significant differences in infectious disease evolution. We investigate the correlation between individuals’ travel behaviour and epidemic diffusion, to quantify the risk of infectiousness/infection of the population at different scales.
As a first contribution, we use the model to investigate the best ways of identifying the most vulnerable geographic areas of the country. We approached it as the identification of influential spreaders within a network of locations. Relying on complex network theory, we compute the risk associated to each node (i.e. location) as a function of its accessibility (the higher the accessibility the higher possibility of interactions). It is determined considering the number of people moving to it, where the contribution of the people is a function of the accessibility of the area they come from and so on.
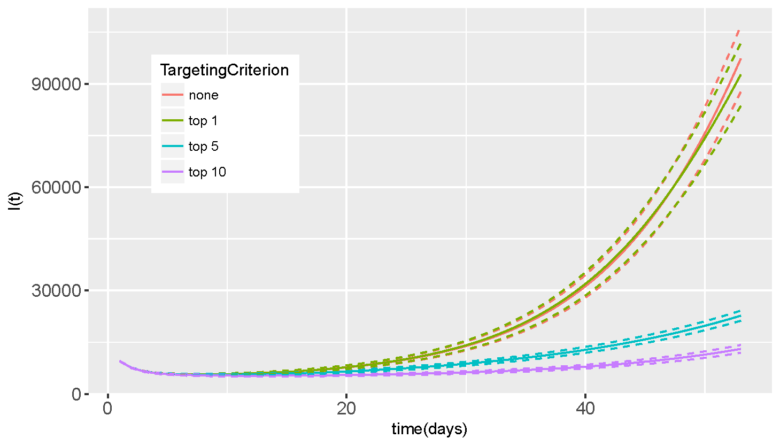
Figure 4 Number of infections when quarantining the highest-risk sub-prefectures
(i.e., top 1, top 5 and top 10 highest ranked sub-prefectures)
Figure 4 shows an example of evaluation of mobility restriction measures on different sets of highest risk sub-prefectures, selected based on their accessibility. The number of cases changes with specific interventions in place.
Going a step further, we considered the infection risk associated to single individuals based on their mobility profiles. Vaccines can prevent outbreaks of disease and save lives, but not everybody needs to get vaccinated. It is in principle sufficient to immunize a fraction of a population (see herd immunity), but this very much depends on the social and spatial behaviour of each individual. Indeed, the higher the mobility of an individual, the higher the probability to get infected, and if infected then to infect other individuals. Hence, a selective vaccination campaign could be set up thanks to the assessment of each individual’s probability of infectiousness based on his mobility pattern.
To this end, we analysed existing mobile phone-based mobility indicators and studied their correlation with the contagion risk of individuals computed in our model. Specifically, we considered three critical perspectives:
- the spatial range, in term of distance travelled by the individual (as measured by the radius of gyration)
- the spatial regularity or the heterogeneity over the sequence of visited locations (as measured by the movement entropy)
- the frequency of mobility (as measured by the percentage of time spent at home).
As an additional index for the quantification of contagion risk, we considered a hybrid risk model, namely Progmosis, which leverages both the mobility behaviour of single individuals and the epidemic dynamics itself.
Once more, the model allowed us to analyse their relevance in identifying the highest-risk sub-population of individuals as the best candidates for isolation countermeasures, like vaccination campaigns. As shown in Figure 5, we could state that, among the others, isolation based on Progmosis risk model presents significant effects on the reduction of the number of infections when isolating at least 20% of the population.
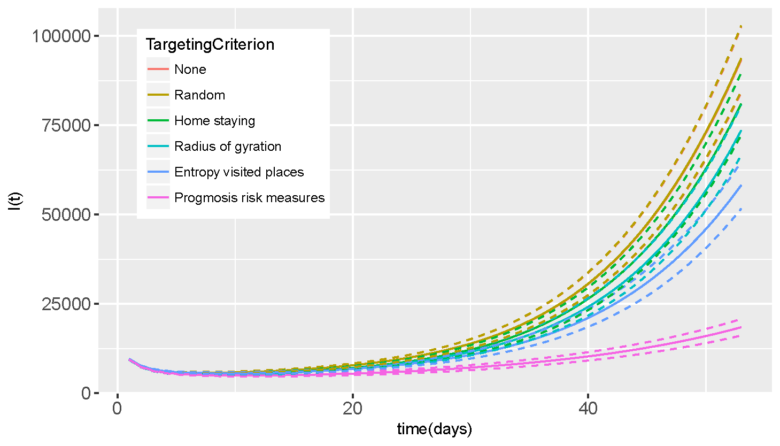
Figure 5 Number of infections when isolating the highest-risk individuals
(i.e., the 20% of the entire population, selected according to their mobility profile, defined by the above described mobile-phone–based mobility indicators)
CONCLUSION
The approaches presented in this paper provide further evidence that mobile phone data can be effectively exploited to facilitate our understanding of disease dynamics, of individuals’ spatial behaviour and its relationship with the risk of disease contagion. We also provide evidence that successfully performing epidemic containment strategies may require the identification of differences in mobility patterns among individuals.
One of the main scientific challenges is the inclusion of the actual social factors into the model. Epidemic spread depends on the individual’s interactions with the external environment, on how his behavior adapts to external stimuli. How does the spread of information about a disease outbreak affect his behavior? And how, in turns, does it affect disease progression? These are all open questions that, while 10 years ago were out of reach, we are now in the position to be able to address.
More info
[1] Salathé M, Bengtsson L, Bodnar TJ, Brewer DD, Brownstein JS, Buckee C, et al. (2012) Digital Epidemiology. PLoS Comput Biol 8(7)
[2] Brownstein JS, Freifeld CC, Reis BY, Mandl KD (2008) Surveillance Sans Frontières : Internet-Based Emerging Infectious Disease Intelligence and the HealthMap Project. PLoS Med 5(7)
[3] WHO Report on Global Surveillance of Epidemic-prone Infectious Diseases
[4] Cell Phones in Africa: Communication Lifeline. http://www.pewglobal.org/2015/04/15/cell-phones-inafrica-communication-lifeline/. Accessed: 2017-06-12
[5] Independent Expert Advisory Group on a Data Revolution for Sustainable Development (2014) A world that counts: mobilizing the data revolution for sustainable development
[6] Rubrichi S, Smoreda Z, Musolesi M (2017) A Comparison of Spatial-based Targeted Disease Containment Strategies using Mobile Phone Data. arXiv:1706.00690