● 3.2 times more effective than the current state of the art, the algorithms could put an end to smurfing, a widely used laundering technique that involves breaking up large amounts into small sums.
● The algorithms, which analyse financial flows represented by graphs, may also be used to optimise profits generated by product bundles.
Money laundering (concealing the origin of money obtained from illicit activities) is a major challenge for financial institutions and regulatory authorities. Every year, between 2% and 5% of the world’s gross domestic product (GDP) is laundered.
Manual methods and machine learning tools to detect the practice have a number of drawbacks, most notably a lack of responsiveness linked to the need to analyse large volumes of transactions. “Until now, banks have used manual systems that are not scalable,” explains Grigorios Loukides, an associate professor in the department of informatics at King’s College London. He further adds that “systems proposed by other researchers do not offer guaranteed of results, which ours does.” In a bid to overcome some of these drawbacks Loukides and his colleague Huiping Chen recently published a research paper detailing algorithms with the capacity to analyse 50 million transactions in 50 seconds, which they designed to combat , a commonly used money laundering technique.
We don’t use machine learning. Instead, we model the data in the form of graphs and then identify areas that are most suspicious
A novel anti-smurfing approach
The technology proposed by the researchers aims to combat a money laundering technique known as smurfing, which involves splitting a large sum into multiple small amounts. Previous research has shown that money launderers are behind virtually all the initial placements that they subsequently expect to recover. Thereafter the new approach also analyses the behaviour of the holders of intermediate accounts into which the money is paid as it slowly makes its way back to the criminals. The method developed by the researchers is said to be 3.2 times more effective than the current state of the art on the market.
“We don’t use machine learning. Instead, we model the data in the form of graphs and then identify areas that are most suspicious,” explains the researcher. “So we don’t need to train a model to detect risks, which is very difficult to do with such noisy data.” Graph modelling also allows the researchers to represent accounts as nodes, and transactions as edges in a complex network.
Possible applications in marketing
Along with money laundering detection, the technology also has potential applications in other areas. For example, it could be used to optimise marketing campaigns by helping retailers select the most profitable product bundles. Grigorios Loukides explains: “We have tested the tool with customer and distributor accounting data, demonstrating its effectiveness in optimising profitable product selections.” Analysis using graph systems can reveal which products sold together offer distributors the greatest potential for profitability.
One of the most common methods of money laundering, smurfing involves breaking up large sums into small cash amounts, which are deposited into multiple accounts to avoid triggering suspicion.
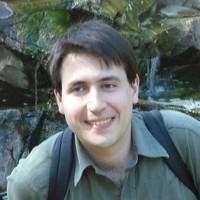
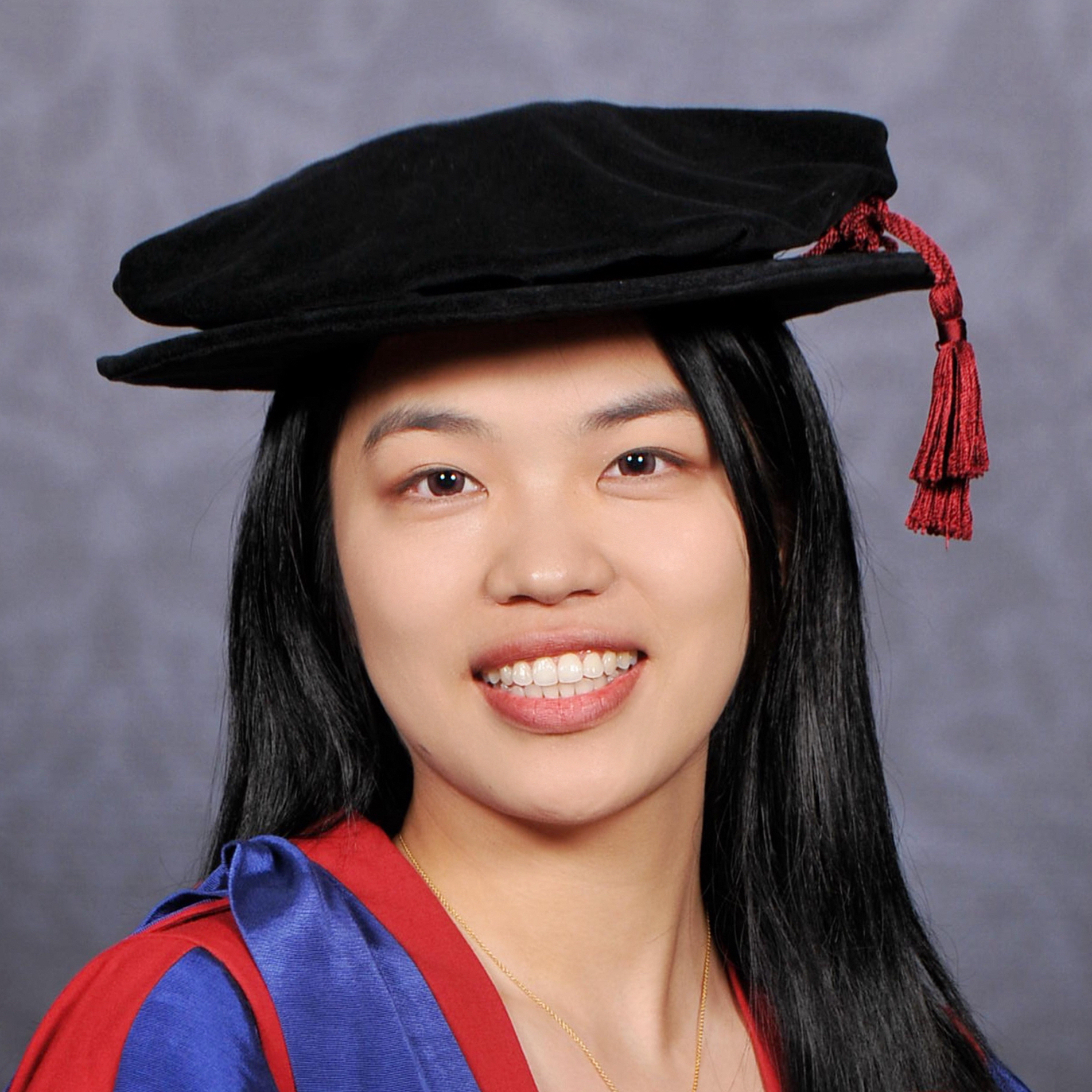