Internet down? Service unavailable? Wi-Fi problem? Various teams at Orange are involved in solving these problems, with the aim of providing its customers with the best possible service. This commitment applies from operational teams to research teams.
Diagnostics are a major challenge for Orange as they help to maintain a high level of customer satisfaction, control operational costs and limit the carbon footprint associated with travel and field interventions.
So, while the Internet starts and stops at the box for the customer, the reality is quite different for Orange and anticipating the resolution of some faults is quite a challenge.
It’s like swimming through a sea of cables!
What’s at the End of the Fiber Cable?
The fixed access network represents the last meters or kilometers of fiber needed to bring the Internet to the customer’s box. But this journey isn’t always smooth sailing. Box, endpoint, branch point, distribution point, splitters, central offices… The data has a long way to travel from the customer to the heart of the network. On top of this, there are additional services such as TV, VoIP or data. In short, it is not easy to diagnose faults when there are so many possible fault sources.
Once the data has been collected, it is processed by a specialized diagnostics system that contains all the knowledge of previously seen faults.
It should also be kept in mind that the network is constantly evolving, whether through changes in network equipment, topology or configuration, or through services and usage, which are evolving as well. The constant changes in the environment generally explain the appearance of new faults, which need to be identified, characterized and anticipated.
Doctor, doctor!
To ensure that a customer’s line is working correctly, Orange can carry out a network health check. Is a customer reporting a problem? A test on the customer’s line collects technical information about the status of their connection and services. This data is really valuable for detecting possible anomalies.
Once the data has been collected, it is processed by a specialized diagnostics system that contains all the knowledge of previously seen faults. The purpose of this diagnostic system is to analyze the data and categorize the fault experienced by the customer. When the system identifies a known fault, it is then easy to implement the best protocol to resolve it. If an intervention is needed, the technician will know precisely where to go or, in some cases, the operational teams will know that there is no need for an intervention and that simply requesting new equipment to be sent to the customer is enough, for example. This can be done directly from the call to the agent.
This saves time, both for the customer who will see their service restored more quickly, and for Orange teams who will be able to focus on more complex interventions.
The Problem with Unknown Faults
However, no system is perfect and there are still faults that cannot be automatically identified at the time. But don’t panic! Even in this kind of situation, Orange teams have protocols that always provide the customer with a solution. The nature of a new anomaly, however, means that restoring the customer’s services will be more complicated and take longer, compared to when dealing with a well-known fault.
As a result, identifying and categorizing unknown faults is becoming a major challenge in better integrating network developments into the diagnostic chain.
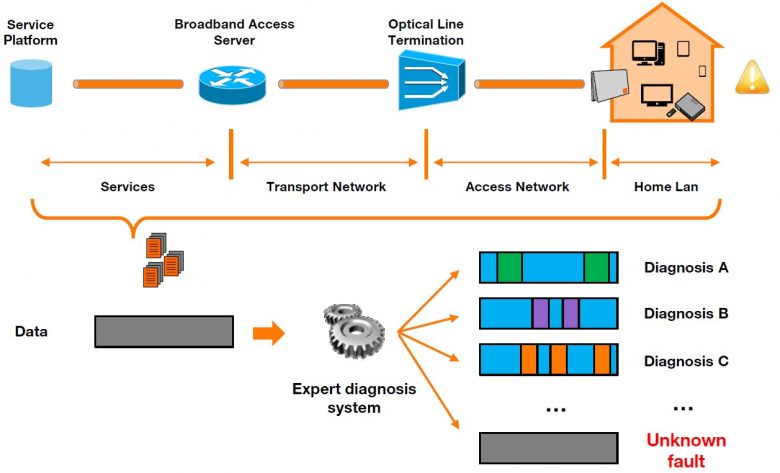
What Is the Role of AI Research in All of This?
Discovering new types of network faults is not easy. Thousands of pieces of technical data need to be analyzed and cross-checked, and currently, no solution exists. This means that the research teams have a lot to think about.
The goal is to identify new fault signatures in customer data for which no known conclusions have been found. In concrete terms, this fault signature means searching for a link between technical characteristic parameters. For example, this can be the appearance of an alarm that would be linked to both the version for a piece of equipment and a given service. In other words, it can sometimes feel like looking for a needle in a data haystack.
The research work is paving the way to develop machine learning models* that are capable of helping to discover new faults. These models are built by using large amounts of technical data from the network.
Here, unlike conventional approaches, the idea is to use the knowledge about known faults to guide the exploration of new faults.
This is the type of approach that we have developed as a proof of concept [Démo Salon de la recherche : https://mastermedia.orange-business.com/publicMedia?t=pmJe7ar1yN] and which has been the subject of scientific publications, at the IEEE ICKG 2022 conference and in the IEEE Access journal.
The programmed machine learning model makes it easier for clusters* of faults to be identified. Each of these clusters can generally be characterized by a specific technical signature.
Continuous Improvement
When a new technical signature has been identified, it is sent to network experts to verify the validity of this preliminary new diagnosis. Because, even in a world where data is everywhere, human expertise remains at the heart of the validation process. For this, a lot of tests are carried out. These tests are all the more important, as the entire diagnostic chain can be impacted by validating a new technical fault signature: agents, operational staff, field engineers, etc.
This process can then be repeated regularly to continuously improve the diagnostic chain (see figure), and make it more resilient to any type of change within the network. This therefore helps to maintain a high customer satisfaction rate, control operational costs and reduce the carbon footprint associated with travel and field interventions by several thousand metric tons of CO2 per year.
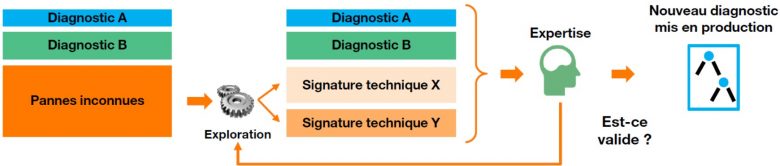