The machine learning
Machine learning is a fast-growing field of application, which constitutes a set of methods, techniques and algorithms that allow complex Artificial Intelligence problems to be solved by learning from a large data set.
It is precisely machine learning that defines the algorithms for recommending movies, music, or even autonomous cars!
But how exactly does a machine learn?
Let’s take the example of house prices in a neighborhood.
We will present the machine with the prices of thousands of houses and their descriptions. Using machine learning algorithms, the computer will automatically learn the characteristics that make a house more or less expensive (surface area, location etc.). This is how the computer will deduce rules.
From this data and this learning, the computer will be able to predict the result, i.e. the price of a house.
Machine learning is an innovative approach because it radically changes the traditional way of coding (a human listing precise but subjective rules on a software that will calculate a result). Here, the machine, from the data at its disposal, deduces by itself adequate rules to answer the task. The choice is therefore theoretically more objective.
It is thus thanks to machine learning that we can create more and more powerful artificial intelligence rules!
Read also on Hello Future
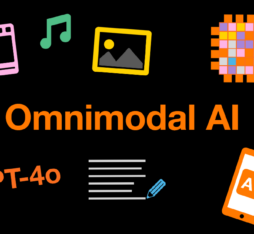
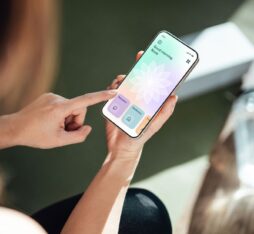
AI therapy: marketing hype and the hidden risks for users
Discover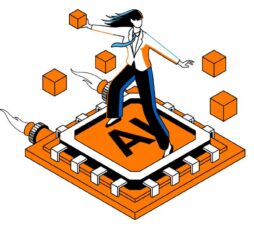
A lexicon of artificial intelligence: understanding different AIs and their uses
Discover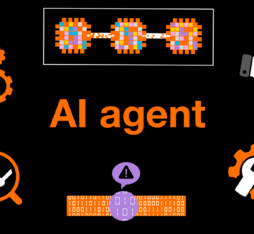
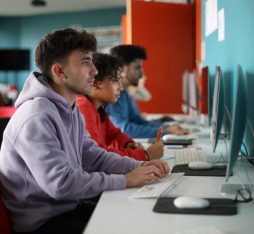
Devoxx France: “AI has ushered in a second revolution in the world of testing”
Discover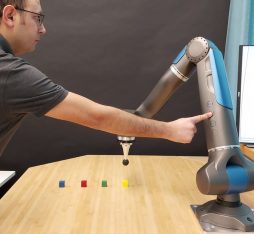
Machine learning for intuitive robots that are aware of their environment
Discover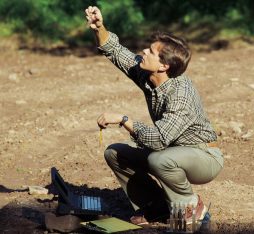
Geology, geoarchaeology, forensic science: AI reveals history in grains of sand
Discover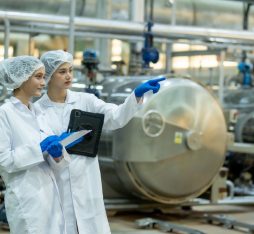