How do Internet users react to this paradoxical proposal of 'speaking' to robots? Do they address chatbots the same way they would a seller or do they ask it questions using keywords, in the same way they would with a search engine? Do exchanges remain purely linguistic or are they supplemented with other forms of screen interaction, like buttons, menus and guided tours? Lastly, what do these interactions between humans and robots look like? The study presented in this article provides some answers thanks to an analysis of interaction logs from the Djingo Pro bot, used on the Orange portal for business customers and companies since 2017.
"The logs highlight a variety of ways to interact with chatbots, moving away from a single model of language interactions."
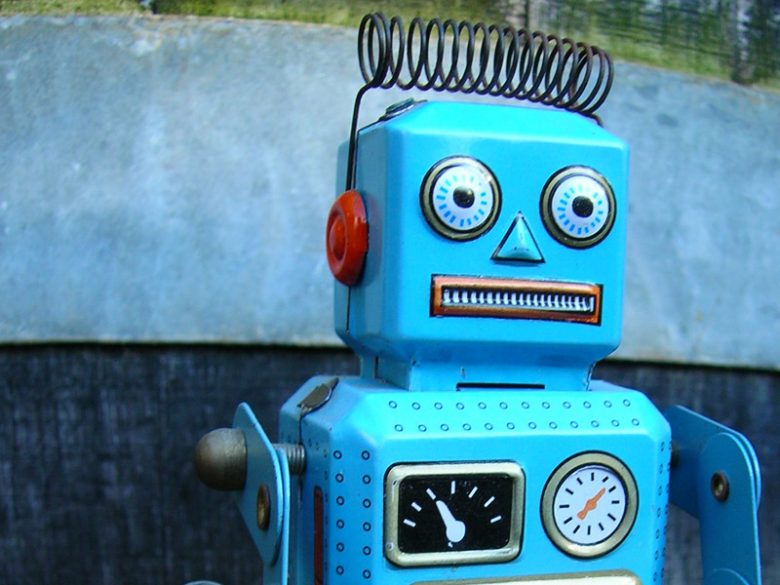
Peyri Herrera – Flickr – CC BY-ND 2.0
Djingo Pro, the support bot for pros
Facebook opened Messenger to bots in 2016 and in doing so marked the rise of the chatbot. Specialist marketing and customer relations publications are interested in this new means of interaction that seems to combine the advantages of automation with the warm and open nature of conversation (see Dialogue with machines: between fantasy and reality). Since 2017, many companies have begun to experiment with this tool to get a sense of its potential and limitations.
Orange is one of those pioneers, launching the development of a series of text bots, or chatbots. These bots are presented to users as instances of a unified, multi-channel conversational interface of the brand.
The ‘professionals’ portal (pro.orange.fr) was one of the first to implement a bot solution, with a first release in September 2017. It handles technical support requests directly through its conversational interface. The first use case involved retrieving a PUK code, which you need if you have blocked your cell phone after incorrectly entering a PIN several times. Subsequently, the functional scope expanded rapidly to include SIM card activation, billing information, general practical information, Internet and landline technical support, as well as routing to an advisor or redirecting to other digital services.
Figure 2. Djingo Pro, 2018
To understand how Djingo Pro users interact with this chatbot, this study is based on an analysis of bot logs from May and June 2018. The dataset studied consists of 2,771 instances of interaction with the bot. These interactions were anonymized and split into alternate bot and user turns-at-talk. Finally, to quantitatively analyze users’ turns of phrase, morphosyntactic labeling was carried out. This is an automatic process that identifies verbs, names, infinitives, genres, etc. in a text. These tools work well on grammatically ‘correct’ texts and in this case, they perform well since the text inputted by chatbot users tended to be ‘well’ written.
Since then, Djingo Pro’s functional scope and user journey have evolved, and ambiguity management has improved; understanding of how the chatbot is used has increased thanks to this study.
Framing conversational interactions
Interactions with chatbots are generally brief. A ‘conversation’ with Djingo Pro in spring 2018 involved an average of 11.3 turns-at-talk (median: 9). For users, this corresponds to an average of 5.1 turns-at-talk (median: 4). The length naturally varies depending on when users end interactions and on the chatbot’s ability to deliver user satisfaction — authentication is a significant sticking point and at the time of the study, almost one in three conversations stopped at this stage.
However, when users receive a response to their need expressed via the bot, two thirds of them respond to the bot’s request for positive or negative feedback, “Are you satisfied with my answer?”, to which almost half say yes and a third stay on until the bot ends the conversation by writing “Have a nice day.” A significant proportion of chatbot users therefore follow the conversational script and respond to requests even after receiving the information they wanted.
While the format of the conversation serves as a framework for the interaction, it is very guided in terms of how it progresses, schematically structured into three stages: expression of need, response to that need and user feedback. At first, the interaction begins with an open question from the chatbot, “Hello, I’m Djingo, your virtual assistant. What can I do for you?” This question lets users freely express their need or situation. An essential part of the project team’s work is dedicated to correctly interpreting this initial request and translating it into a formalized need, a series of detailed solutions, and a varying amount of time to achieve it, especially when users have to prove their identity.
In the bot’s technical system, this mechanism is presented as a list of ‘intentions’—to which the bot can respond—and ‘variations,’ a list of user expressions that may correspond to these intentions. The project team is constantly reviewing interaction logs with the aim of identifying these forms of expression in order to improve how effectively intentions are recognized.
Therefore, once chatbot users have expressed their situation, the rest of the interaction is guided by the script for the recognized intention. During this second stage, the interaction relies on users’ free expression as little as possible, instead offering users buttons to limit the range of responses as much as possible.
Using answer buttons acts as a way to improve service efficiency by clarifying what answers are expected. It also helps to reduce the burden of the interaction for users, who do not have to type out text but can just choose from options.
In the third stage of the interaction, the chatbot asks users for their feedback, for which the possibility of entering free text is restored, but this time it is not interpreted as an intention[1].
This three-stage process of interacting with the bot translates in practice to a high prevalence of button responses in interactions. The majority (48%) of users’ turns-at-talk were button actions, 9% involved entering credentials for authentication purposes, and only 42% were ‘natural’ written text, i.e. an average of 2.2 turns-at-talk per conversation.
Types of turn-at-talk by users
Users were often brief in their natural wording — on average, they wrote 8.8 words (median 6, max. 141): “I need my PUK code,” “password reset code,” or “Hello I need to unlock a cell phone.”
Six ways to interact with a bot
How users formulate their first interaction with a chatbot is therefore crucial for how the interaction unfolds. It is the most open request for expression, and the most essential to the success of the interaction. How do chatbot users express themselves at this particular moment? Can they formalize an explicit need? Do they bother to write full sentences?
There are two contrasting factors that structure modes of expression:
- keywords (32%) vs. sentences (68%): two-thirds of needs are expressed as a sentence containing a conjugated verb, such as “I want to activate my SIM card.” In other cases, short forms are preferred: “PUK code,” “activate SIM card.”
- need (65%) vs. situation (35%): 65% of users formulate an explicit need in line with the range of services offered by the chatbot: “I need my PUK code,” “activate SIM card.” Meanwhile, other users describe their situation and expect the tool to provide them with suitable solutions: “I’ve blocked my cellphone,” “lost password.”
Six ways of expressing a need
The way these two factors cross over and carefully examining the formulations have helped establish six major ways in which chatbot users express their needs.
In short forms of expression, the Google search method (“PUK code,” “need PUK code”) seems to be in the minority (11%). Meanwhile, robotic commands recognizable through the use of infinitive word phrases (“activate SIM card,” “get PUK code”) accounts for almost one in five (18%). These short forms are widely used by users who have a precise idea of what they are expecting from the bot, but they are used much more rarely (3%) to briefly articulate a situation.
The other three forms of expression follow the conversational format suggested by the chatbot: users write grammatically complex sentences, of around ten or more words, in the first or third person. Three subgroups stand out. Firstly, expressing explicit needs (28%) or obstacles (7%) corresponds to situations where users know exactly what they expect from the chatbot, identifiable by the use of verbs of intent or verbs of incapacity: “I need my PUK code,” “I can’t activate my SIM card.” Finally, in a third of these interactions, users provide detailed accounts of the situation (32%): “I have blocked my SIM card,” “how do I unlock my daughter’s phone, which is on my pro account?”
In spring 2018, these accounts of situations and descriptions of obstacles proved the most difficult for the bot systems in terms of matching a user’s expression to the list of expected and coded ‘intentions.’ Where references to “PUK code” and “activate SIM” are easily recognizable, the plethora of individual situations and possible solutions makes it more difficult to translate the original expression into a formal customer need from a company perspective. Building on these study results, the project team has been working to include these types of expressions in the chatbot’s supervised learning.
Toward targeted log monitoring
The logs highlight a variety of ways to interact with chatbots, moving away from a single model of language interactions. For example, one third of chatbot users do not engage in conversation, preferring to use keywords as they would when using a search engine. However, another third of users express themselves with fully formed sentences, or even detailed accounts. If the initial request is formulated in a textual manner, half of the interactions are not even text, but clicked buttons: There is therefore no typical user, but a multiple number of uses and possibilities for using the interface.
This typology therefore shows that chatbots do not offer a unique model of interactions, although framing conversations remains crucial for the use of the interface. This diversity of uses enabled the Djingo Pro chatbot project team to examine these different interactive modes, in order to pay particular attention to the more ambiguous forms of expressions to be handled. Patrice Anglard, project Product Owner, explains that these results made it possible to better address the diversity of user expressions and to propose targeted solutions: “Users’ overly vague or confusing requests can be handled by ‘automatic disambiguation,’ but Djingo Pro also encourages users to express themselves in full sentences when they only use a few keywords.”
If users write sentences that are too long, the interface will suggest a simplified rewording or offer multiple-choice answers. Conversely, when expressions are too short for the chatbot to distinguish between several answers, it suggests that users write a complete sentence. By taking these two modes of expression into account, the interface can both understand a greater diversity of language and get users to express themselves in a more standardized way when these modes of expression verge too far from Djingo Pro’s understanding model.
Natural language processing can only operate within a somewhat broad anticipation of the needs expressed by users. While the project teams developing chatbots continuously analyze natural language and user interaction, a targeted analysis of users’ modes of expression helps to gain insight into how these tools are used and, in this case, to improve how they work with solutions based on actual uses of the tool.
[1] The ability to enter feedback as free text has now been removed because there was not much feedback being entered, with most texts being reformulations of the request.