Recent advances in automatic speech processing – notably through the improvement of some deep automatic learning techniques [1], made possible by the availability of increasing volumes of “training” data and larger computing resources – have led to the development of a new generation of “intelligent” voice assistants (IVA).
Promotional discourse on IVA (personal as Siri and Google Now or domestic as Amazon Echo or Google Home) often emphasises their use of “natural” language or dialogue. By design, however, their modus operandi is based on a two-part voice command (user’s request + system’s response), preceded by an activation word (e. g. “Ok Google”).
In the home, systems like Amazon Echo and Google Home process a wide variety of verbal instructions to access information (e.g. weather, traffic) or multimedia content, dictate text messages or control household appliances (heating, lighting, TV, radio, security devices, etc.).
The use of these systems raises a number of questions about their use: What is the nature of people’s interactions and relationships with these assistants? How do families appropriate them, and for what purposes? What benefits can the current assistants bring to a home?
To shed light on these questions, we conducted two usage studies in the home environment, based on video observations and interviews. The first involved three English-speaking families who are asked to test two systems (Ivee and Amazon Echo). The second concerned an experimental prototype called “Alice”, developed as part of an orange research project and tested by seven families in 2016-17 (Fig. 1). The usage of these systems ranged from 3 weeks to 4 months, depending on the families.
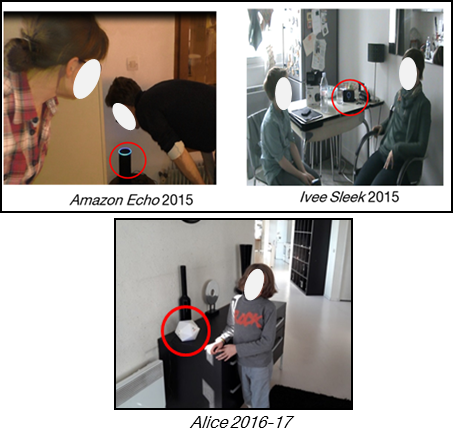
Fig. 1 – Voice-activated “intelligent” assistants studied and usage situations.
The adaptation process
“Natural” interaction – fluid, effortless and very close to human conversation – is the promise made in the promotion of intelligent home assistants. Yet our studies show major discrepancies between that promise and the reality of the interactions observed.
Above all, speech recognition is not always effective, including for simple requests like the following (U = User):
Example 1 – Speech recognition failure
1- U : hello ivee what time is it in New York City?
2- Ivee : it is now 4:41 am in Sydney Australia
Users sometimes have to repeat their questions several times to make themselves understood, which can lead them to give up on the system very quickly. If they do persevere, they engage in a process of “repairing and making sense of the interaction” [1]. This repairing work may involve a variety of actions, such as reformulating their statements by shortening them (see example 2) or clarifying them with further details, moving closer to the device, or talking more loudly.
Example 2: Syntactical adaptation (reduction) of the command
Transcription conventions:
[ = Time overlap between two events
(.) = brief silence1- U: (.) Alexia go on to YouTube and look up Katy Perry please
2- Alexa : hm (.) I can’t find the answer to the question I heard
3- U : Alexia (.) go on to You Tube (.) now
4- Alexa : hm (.) I can’t find the answer to the question I heard
5- U : Alexia You Tube
These efforts go beyond the wording of the commands, encompassing all activities people engage in to get the system to work, including making sense of the system’s inappropriate responses, or learning about the interactional structure imposed (activating the system, then speaking at the right time, when the device is “listening”).
Finally, the effectiveness of this adaptation work varies from person to person. We have observed, for instance, that young children have great difficulty making themselves understood, which creates a sense of exclusion and generates frustration and resentment. Today’s voice-activated systems thus require a process of adjustment, both individual and sometimes collaborative (in the form of mutual assistance), from members of a household (see example 3 below) as well as guidance and support for children.
Contrary to the promise of fluid, “natural” interaction, this adaptation work is in fact typical of all interactive voice response systems. It may take various forms depending on the context and the type of voice interface (see [2] for a study on Interactive Voice Response systems in Orange customer relationships). When it takes too much effort, however, it may prevent users from appropriating the system, or even cause them to abandon it.
The illusion of a natural conversation
The ability of voice-activated intelligent assistants to manage dialogue in a fluid manner is at the heart of the promotional discourse surrounding them. In reality, however, that ability is limited, producing the appearance of a natural conversation while actually generating interactional difficulties.
When the systems function optimally, users tend to implicitly ascribe capabilities to them that they do not actually possess. An example is the use of indexical terms (such as “now”, or “here”), which refer to contextual elements that the systems are incapable of processing. This is what we can see in example 3, where the first user (U1) refers in line 5 to an element mentioned previously (“it”, which refers to what is said in line 1, “cooking pork”). We should note, in passing, the speech recognition problem manifested in Alexa’s response in line 4 (the system adds what U1 has said to a shopping list), which leads U1 to rephrase her request. Interestingly, we can see that U1 repeats the referent “it” used by the machine (line 4), which may suggest that the machine “understands” the meaning of this kind of indexical term. But this repetition creates difficulties.
Example 3: Use of indexicals (“it” in this example)
1- U1 : Alexa I [need a r, I need to cook some pork
2- Alexa : [feed-back lumineux
3- U1 : [and-sh
4- Alexa : [I added it to your shopping list
5- U1 : and I need a recipe for it can you find me one?
6- U2 : You didn’t say Alexa
7- U1 : Alexa need I need a recipe for it can you find me one?
8- Alexa : I was unable to understand the question I heard
Subsequently, the person forgets the activation word (line 5), a problem that is pointed out by another family member, U2 (line 6) and that U1 then corrects (line 7). But Alexa is unable to provide the expected response, probably because it is incapable of interpreting the indexical ‘it” repeated in line 7. This incapacity is one of the biggest challenges for Artificial Intelligence: understanding the context. However, the reason for this failure is never explained, and remains unclear for the users involved in the interaction. They thus find themselves in a paradoxical situation with regard to intelligent assistants: the better the system works and the more it uses ordinary conversational practices such as the use of indexicals (“it”, here), the more users tend to speak “naturally”, and the greater the risk of the dialogue failing without the users being able to determine the cause of that failure. In other words, these systems create the illusion of a natural conversation, and users are not fully aware of the limits of the systems’ comprehension capabilities.
The question of trust
These assistants are “listening” constantly so as to be able to detect their activation word. This is a source of concern. Users have no way of knowing what is really being listened to, processed and stored. They see these virtual assistants as “black boxes” with opaque inner workings of which they need to be wary. As the interview extract below suggests, greater transparency on how the system works, as well as the possibility of controlling it, seem to be important features for users.
“It should be clearer when you can disconnect and reconnect. It should be possible to have times in a day where you can say “OK, Alice is sleeping. She’s gone to sleep and she’s not doing anything. She shuts off. She disappears from your life.” (extract from interview – Alice study).
It is interesting to note that this lack of transparency has become a public concern. For example, various actors (institutions, academics, etc.) have proposed recommendations for use as privacy protection measures. This is for example the case of the CNIL, which is an independent administrative authority aiming at protecting the consumer against any misuse of her/his personal data [3].
Conclusion
In addition to the identified limits of voice-activated home assistants, our studies also highlight their potential usefulness as a unified voice interface for accessing all domestic and multimedia appliances. In general, participants saw their potential benefits despite the sometimes erratic quality of speech recognition.
However, our analysis also highlights the considerable adaptation work required from users of today’s intelligent assistants, work which could compromise long-term adoption if it requires too much effort. Optimizing speech recognition and dialogue management and minimizing the amount of effort required from users are therefore crucial avenues for improving these systems. Furthermore, despite recent progress in artificial intelligence, the ability of these machines to provide a truly “natural” interaction remains limited. To avoid users developing excessively high expectations, it is important to talk more realistically about their current capabilities.
Several areas for improvement emerge from our analysis, concerning the intelligibility of conversational technologies. The first is enabling the user to understand the system’s answers and the source of the problem if the system gives an inappropriate answer or is unable to respond at all. The second is guiding them to help them formulate their commands effectively (e.g. by giving them examples of how to formulate a particular command). Another area is to improvement dialogue management by developing systems that are capable of making “repairs” like those found in human conversation [4]. These are the processes through which speakers deal with difficulties that emerge in interaction (for example, requesting clarification of a misunderstood statement, repeating a statement that has been misunderstood). This presupposes that IVAs are no longer limited to managing two conversational turns (one command and one response) as they are today, which presupposes an ability to build a larger history of the interaction.
In addition to improving IVAs’ interactional capabilities, other, equally important challenges have to be addressed. For example, managing access to the system according to the speaker’s identity is a crucial point, as without authentication, a voice assistant raises a number of security and privacy issues. On this point, one of the solutions explored is speaker recognition, but some problems remain, such as the intra-speaker variations. Current AVIs such as Google Home and Alexa can learn to recognize a user’s voice but this technology is not robust enough in terms of security [5]. The untimely activations of AVIs, that is, not initiated by individuals, also raise security concerns. For example, recently, the Alexa system recorded and sent a conversation extract to a random contact of a couple without their knowledge [6]. This raises the problem of user control and also trust. The privacy issue also arises in relation to the service provider. Concerning this point, as we have shown, users do not have a clear understanding of how these systems work (what is recorded, stored, etc.), which generates mistrust. A central challenge for companies offering these devices is to ensure that the personal data collected (household members’ conversations and activities) is protected, while offering the people concerned visibility and control over that data. Addressing all of these issues – enhanced dialogue management, intelligibility, control, security, privacy and trust – is likely to play a crucial role in the adoption of these systems.