PINNs
The inventors of PINNs define them as “neural networks that are trained to solve supervised learning tasks while respecting any given law of physics described by general nonlinear partial differential equations”.
Indeed, algorithms do not necessarily take into account the physical principles governing the systems to which they are applied. Yet, the behaviour of these systems is dependent on different fields (such as mechanics or thermodynamics) where each has its own laws and models, which constitute a precious source of information.
Consequently, even the most advanced techniques of machine learning are sometimes not very efficient at solving complex scientific and engineering problems.
The idea of PINNs is therefore to “encode” the laws of physics and scientific knowledge into learning algorithms so as to make them more robust and to improve their performance.
According to their inventors, the addition of this crucial information can restrict the field of possible solutions, which would enable the algorithms to aim for the right solution faster and to become better at generalising, meaning to function correctly in the real world, with data that they have never seen before.
PINNs are of interest to the world of research in a wide range of areas including climatology, seismology, or material science.
This approach is also of interest to industry. For example, to create the digital twin of an aeroplane, simulation software using PINNs will take all physical phenomena into account as well as their interactions with one another. It will therefore integrate the rules of aerodynamics and mechanics that make a plane fly, as well as general data throughout its lifecycle.
Read also on Hello Future
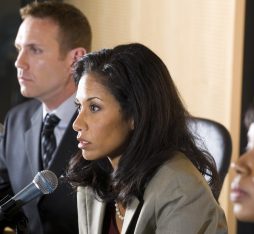
Controversies around AI: from ethical questions to legal regulation
Discover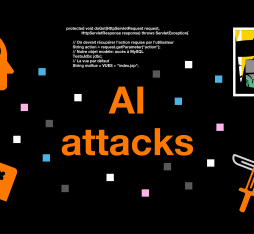
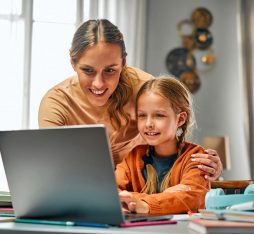
Ethical AI and children: the benefits of a multi-disciplinary approach
Discover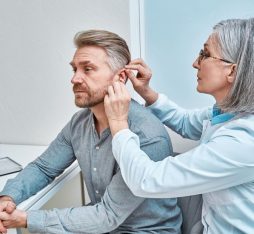
Neurotechnology: auditory neural networks mimic the human brain
Discover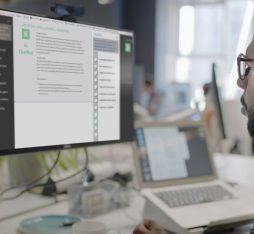
P-C. Langlais (PLEAIS): “Our language models are trained on open corpora”
Discover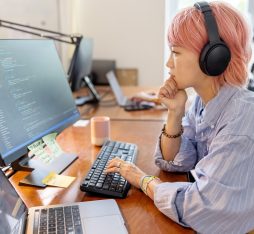
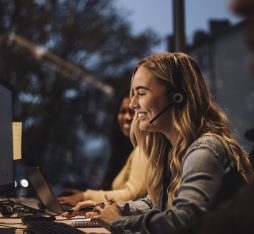
FIND, a Search Tool That Uses Generative AI to Answer the Pros’ Questions
Discover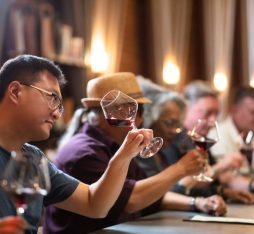