• A research team at the University of Zurich has been working on the integration of these new devices in driver assistance systems, which should pave the way for faster obstacle detection in autonomous vehicles.
• Event cameras, which continually capture changes in brightness on the level of individual pixels, benefit from vastly reduced data flows and storage requirements. In China, a research team has recently announced the development of a vision chip that can capture up to 10,000 images per second.
Imagine a new generation of cameras that consume up to 100 times less energy while transmitting image data at 100 times the rate achieved by current devices. These are just two of the game-changing properties of bio-inspired, neuromorphic or event cameras, which could soon have major impact in a host of applications. Instead of recording a fixed number of frames per second, the new devices asynchronously measure brightness changes for individual pixels while transmitting no data for others that remain unchanged, which leads to a huge reduction in bandwidth. “Elements in the data stream are referred to as ‘events’ because only fractions of the signal are measured by specific electronic chips,” explains researcher Daniel Gehrig of University of Pennsylvania’s General Robotics, Automation, Sensing and Perception (GRASP) Lab.
The research team set itself the goal of combining a neuromorphic camera with a decision-making algorithm without incurring any loss in performance
“In cameras of this type, like those developed by the French company Prophesee, the pixels are continuously exposed but only measure changes in luminance, which effectively allows for continuous signal monitoring.” Put simply, no movement can escape detection by the sensor. “The speed of the camera is equivalent to 5,000 images per second. Any changes will be registered within 0.2 milliseconds, which makes it 100 times faster than a traditional camera.”
Reducing driver assistance reaction times
A few weeks ago, when he was still working in the computer science department of the University of Zurich, Daniel Gehrig published an article in the journal Nature outlining how event cameras could be used to enable vehicles to detect obstacles like pedestrians and cyclists more rapidly. Vehicles equipped with advanced driver assistance detection systems that make use of traditional cameras, which still need to be made faster and more reliable, currently collect around ten terabytes of data per hour.
The Zurich research team set itself the goal of combining a neuromorphic camera with a decision-making algorithm without incurring any loss in performance. “Conventional algorithms analyse images as a whole, unlike the algorithm we developed to process event stream data, which is 5,000 times more efficient in terms of the time required to produce results.” However, to ensure the overall accuracy of the system the researchers also added a second conventional camera at a mere 20 frames per second: “Neuromorphic cameras capture movement, but not the whole scene. Adding a conventional camera gives us context on the vehicle’s environment.”
A Chinese chip that captures 10,000 images per second
For the research team, the next step in this project will be to link their system to a LiDAR. “As it stands, cameras can capture changes in a scene very quickly, but are unable to apprehend distances between objects. The LiDAR will give the vehicle more information and enable it to know how much time remains before it must make a decision.” Ideally, the team would also like to integrate the new algorithm directly into neuromorphic sensors for the automobile industry. However, as Daniel Gehrig points out, “To do this, the algorithm will need to be simplified.”
The Swiss researchers are not alone in developing bio-inspired cameras for intelligent and autonomous vehicles. In China, researchers at Tsinghua University’s Center for Brain Inspired Computing Research (CBICR) have published details of a vision chip called Tianmouc, capable of capturing 10,000 images per second while reducing bandwidth by 90%. Their goal is to avoid data bottlenecks and enable autonomous systems to handle various extreme events with hardware technology that can match the rapid progress of artificial intelligence.
Sources :
Gehrig, D., Scaramuzza, D. Low-latency automotive vision with event cameras. Nature 629, 1034–1040 (2024). https://doi.org/10.1038/s41586-024-07409-w
Yang, Z., Wang, T., Lin, Y. et al. A vision chip with complementary pathways for open-world sensing. Nature 629, 1027–1033 (2024). https://doi.org/10.1038/s41586-024-07358-4
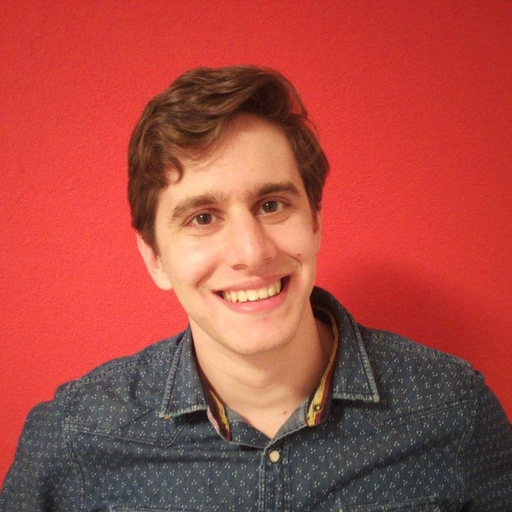