• The team combined data from tastings in which 256 participants grouped samples to reflect similarities in flavour with label information and consumer reviews to create their new algorithm.
• Their novel approach, which could easily be applied to other products, notably coffee and beer, also has potential in healthcare where it could be used to recommend food products and recipes adapted to the taste preferences of individual patients.
Can you predict that you will actually like a wine you have never bought before? There are applications that allow you to read reviews by other consumers along with information that appears on labels. However, they might still leave you in the dark as to what your own perception will be. Now a team of researchers based at the University of Copenhagen has demonstrated that machine learning can be used to achieve a new level of precision when predicting individuals’ wine preferences. “We worked with the Vivino application, which collects consumer reviews and detailed information that appears on bottles, to create a database of wine tastes and their similarities. Our aim was to create a map of flavour impressions,” explains Thoranna Bender, who led the study under the auspices of the University of Copenhagen’s Pioneer Centre for AI. The study’s main focus was the creation of WineSensed, a vast multi-modal dataset, that links information from labels and text from reviews with consumers’ perceptions of flavour. To collect the data for this final element, the researchers organized extensive wine tastings.
An automatic computer vision system analysed spatial relations in flavour annotations to create matrices representing similarities among wines
A study of human impressions of flavour supplemented by machine learning
The researchers combined data collected by the application Vivino, which curates more than 820,000 reviews, with surveys of human tastings: “We used a technique known as napping, which involves grouping wines that people find to be similar. In the course of several blind tastings, 256 participants were asked to comment on shot-sized cups of wine and to position them on an A3 sheet so that those with similar flavours were nearest to each other.” The surveys recorded a total of 5,000 distances between flavours with participants tasting a maximum of five vintages to ensure against palette fatigue. “We then digitized the participants impressions by photographing each of the A3 sheets.” Thereafter, spatial relations in these images were analysed by an automatic computer vision system, which created matrices to represent similarities among the wines.
The WineSensed dataset consists of a large collection of images, user reviews, and metadata about vintages (upper left). In a large user study, we collected flavour annotations of over 100 wines using the “Napping” method [Pagès, 2005], where participants were asked to place wines on a sheet of paper based on their perceived taste similarity (lower left). We propose an algorithm to combine these data modalities into a shared representation (right) and find that using taste annotations as an additional modality improves performance in downstream tasks.
Compiling the results
To obtain a detailed cross-referenced analysis of the results, a multimodal learning approach was used, which combined annotations from the study’s tasting events, information present on bottles such as regions of origin, alcohol by volume percentages and varietals, as well as reviews from Vivino. To connect images with text, the researchers made use of a neural network christened CLIP (Contrastive Language-Image Pre-training), which learns visual concepts from natural language supervising. CLIP was combined with another tool that analysed annotations from tasting participants to establish relationships between these 2D representations and metadata on the studied wines. “Using this method, we were able to represent a ‘flavour space’ for wine, in which the distance between individual bottles represented their similarity in terms of taste.”
The algorithm created by the researchers proved to be capable of predicting consumers’ wine preferences more accurately than other tools that only use traditional types of data in the form of images and text, an achievement that was only made possible by the innovative inclusion of human sensory impressions. It may be early days yet for the novel approach adopted by Thoranna Bender and her team, but their findings, which will likely be of major interest to the food industry, could pave the way for further research that aims to elucidate our understanding of taste. As Bender points out, the method adopted by the study could very easily be applied to other mass-market products, most notably coffee and beer.
Read more :
No level of alcohol consumption is safe for our health (World Health Organization)
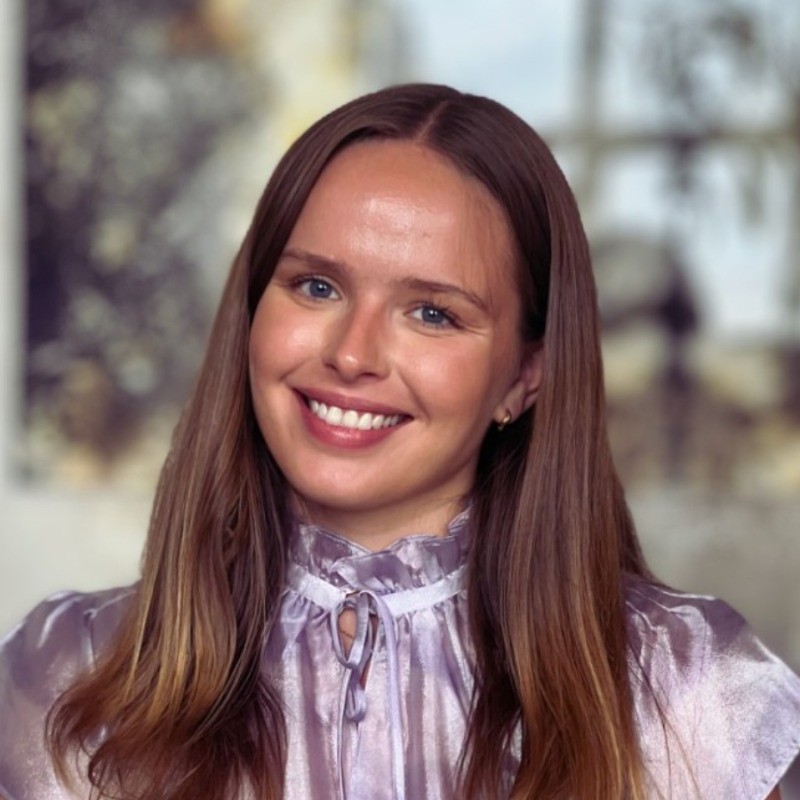