• In Japan, a research team has developed an on-board AI that can locate the voices of earthquake victims hidden under rubble by filtering out noise generated by drone propellers.
• In India, swarms of drones are being programmed to quickly delimit the geographical scope of wildfires, reducing the average extent of burned areas by 65%.
In the wake of natural disasters, or even while they are ongoing, unmanned aerial vehicles (UAVs) or drones can be deployed over affected zones to guide rescue teams and define priority locations for urgent intervention. With their on-board cameras, they provide essential information on damage on the ground, but they are as yet unable to detect people buried under the rubble of collapsed buildings, which is a vitally important task in the aftermath of earthquakes. In a bid to address this critical need, Professor Chinthaka Premachandra, who specializes in artificial intelligence, image processing and robotics at the Shibaura Institute of Technology of Tokyo, has developed a technology to enable UAVs to detect the sound of human voices, which will “improve the efficiency of victim detection in disaster stricken areas and also contribute greatly to our ability to amplify audible signs of life and distinguish them from other sounds.”
Human voices present in audio captured drones can be detected once the noise of the machines themselves has been filtered out by AI
Onboard AI to cancel out machine noise
At the heart of the innovation developed by Premachandra and his team is an artificial intelligence noise suppression system that filters out UAV noise. “It is composed of two new neural networks, a generator and a discriminator, which are set up in competition to form a generative adversarial network (GAN). The generator, which has been trained on real audio of drones in operation, is used to generate pseudo-noise that mimics sound collected by the drone’s onboard microphone, which is then subtracted from incoming recordings, effectively cancelling out the sound of the drone.” Following this process, human voices that would have otherwise been inaudible can then be detected. “The long-term goal is for the generator to produce audio that is so convincing that the discriminator will no longer able to distinguish it from the real thing. However, the process is already generating high-fidelity artificial drone sound.”
To ensure that that the artificial sound is as close to reality as possible, GAN training must be carried out for each specific drone model. “This task can be accomplished efficiently if the training is carried out by the drone’s on-board computer.” For disaster victim detection, the researchers plan to integrate a loudspeaker into the drone. “The on-board speaker relays a message to victims at the disaster site, inviting them to respond. The onboard microphone records the audio outputted by the loudspeaker, but this will also be cancelled out by the system to ensure that it does not hamper victim detection,” explains Chinthaka Premachandra.
Cooperative exploration to combat forest fires
The scientists from the Shibaura Institute of Technology in Tokyo are not the only team investigating the use of drones for disaster management. In Bangalore, researchers at the Indian Institute of Science have developed an algorithm to enable swarms of drones to make autonomous decisions in response to the potential spread and scale of wildfires. Conducting cooperative information-driven searches, the drones, which are equipped with thermal sensors, can quickly locate burning vegetation, and delimit the geographical scope of affected areas. The researchers report that when tested on probabilistic models, “the proposed method reduces the average forest area burnt by 65% and mission time by 60% compared to the best result case of [other] multi-UAV approaches.” Playing a key role in this dramatic increase in performance, individual drones communicate with the swarm indicating the direction in which their peers should continue searching. At the same time, the system is devised to ensure that there is no overlap or redundancy in tasks attributed to each of the drones.
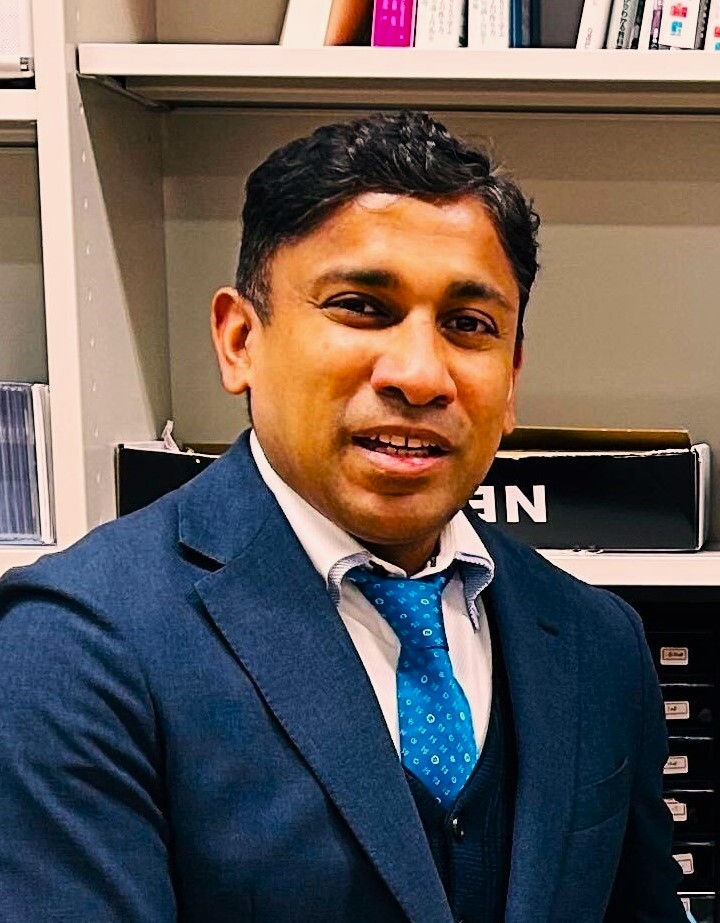