Are we all “addicted” to our screens? A socio-historical look at how digital technology has been pathologised through the prism of addiction.
Are we all “addicted” to our screens?[1] Behind this line of questioning in the media is a deep social concern, a new form of moral panic in the history of communication technologies. Our ability to manage our use of digital technology is coming under increasing scrutiny in social discourse. Orange is committing to raising awareness and controlling screen time with the “For good connections” initiative. This article has drawn on documentary research (specialised literature and public reports) to provide socio-historical insight into Internet addiction.
Read the article

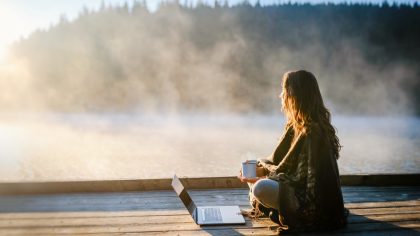
Deepfakes: detection methods struggle to make limited progress
Read the article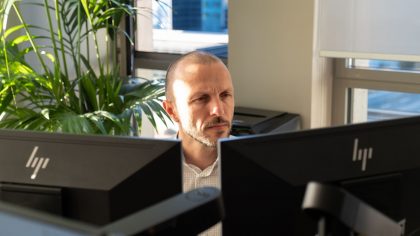
Improving the security of information systems with digital twins
Read the article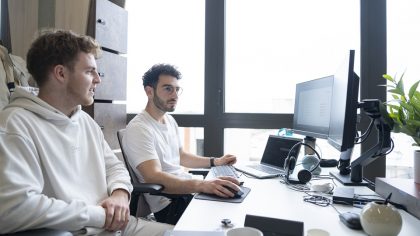
Generative AI: a growing threat to information systems
Read the article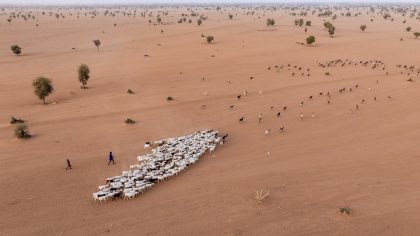
Leveraging Mobile Phone Data to understand Temporary Migration in Senegal
Read the article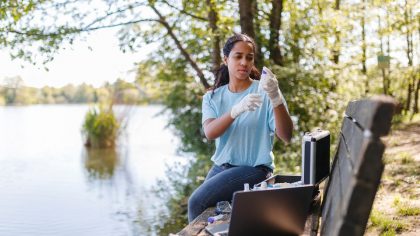
Biodiversity in lakes: multimodal AI crunches eADN data to monitor pollution
Read the article