• In France, AI and cryptography experts working on Inria’s Back In Time project are unlocking the secrets of encrypted historical documents that will soon be accessible to researchers.
• Innovation made possible by AI is accelerating the pace of research in human sciences and encouraging researchers to rethink methods used for the analysis of historical sources.
In the UK, Katherine McDonough a historian and researcher and at the University of Lancaster and the Alan Turing Institute is working on a unique project: a computer vision tool for the semantic exploration and processing of historical maps, which enables users who are new to AI to generate structured data on the information they contain. “We made MapReader because we were very interested in finding new ways to think about historical landscapes, and that led to the creation of a tool that can automatically extract data from historical maps,” explains the researcher. The goal of the software is not only to deepen knowledge of maps and the history of maps, but also to improve understanding of what maps tell us about the history of environments, landscapes, and societies. “Geographic information systems have typically been used to manually trace points, lines, polygons etc. With Mapreader we took a step back from a pixel-level approach to divide maps into different patches that become the unit of analysis. So people can then ask the system: are there trees in this patch, or water, or railway infrastructure etc?” The tool was specifically designed to make it very easy for anyone to create their own annotations, and, if they make mistakes, to modify them without having to start all over again.
We are now seeing more acceptance, greater confidence, and a growing enthusiasm among historians for AI.
New approaches to historical sources
MapReader makes it possible to find specific information at different scales, whether national or international, and to gain greater access to historical knowledge that has not previously been available in this way. “For example, in the past, people in Britain used to play a lot of curling on special ponds with square corners that were specifically dug for this purpose. Using Mapreader, a historian was able to examine how the presence of these ponds in different regions evolved over time, which highlights the historical influence of climate change.” For Katherine McDonough, working with AI is not just a matter of appropriating “off-the-shelf” computer tools to conduct humanities research but of “rethinking methods from another discipline so that they are better suited to answering humanities questions.” She believes that there is more acceptance, greater confidence, and a growing enthusiasm among historians for AI, which they now see as a technology they can adapt to their needs. “If historians are to use AI responsibly, we cannot be content with standard tools. We need to be involved in the design process, otherwise the lack of transparency about how data is transformed or inferred is too problematic.”
Unlocking the secrets of encrypted texts
In France, researchers working on the Inria Back In Time project, which has brought together experts in history, natural language processing (NLP) and cryptography, are planning to unlock the secrets of encrypted historical documents that can no longer be read. “Our dream is to create an online portal where researchers can upload scans of these documents to transform them into readable text,” explains Cécile Pierrot, a research fellow specializing in cryptography at Inria Nancy. However, automation on this level is not on the cards just yet. “Cryptography dates back to 1500 BC and we now have a wealth of historical texts that have yet to be deciphered. Today, we need to automate the process required to read them.” To this end, the team is hoping to create a system that can, for example, generate correspondence tables for sixteenth century documents from scratch. Work to decipher these documents for which the encryption keys have been lost involves the statistical analysis of symbols: how frequently they are used, their positioning and the associations between them, etc. “Today we use optimization algorithms to find keys that will allow us to read the text. We then attribute a score to each of these, and we end up with a graph and a 3D landscape with thousands of possible keys. From there, the goal is to determine the key with the highest value.”
Considerable linguistic challenges
This task is made all the more complicated by the fact that spelling in the French language has not always been fixed. “For example, there are abbreviative symbols with multiple meanings, including one that looks like a ‘9’, which can be interpreted as ‘Con’ or ‘Com’ or ‘Usse’ when it is at the end of a word. Additional natural language processing work was required to deal with all of these,” points out Thibault Clérice, who supervises research on NLP and computational humanities. The recognition of handwritten text in manuscripts adds a further level of difficulty. “Sometimes we don’t know what type of characters we’re going to find in a document, which is challenging for AI both in terms of computer vision and natural language processing.” Nonetheless, the researcher believes that artificial intelligence is playing a very important role in widening the range of historical corpora. “We are now coming to the end of projects focusing on non-encrypted digitized corpora, which is why we need to move on to explore new types of sources.”
Visual: Deciphering Charles V’s letter – Cécile Pierrot at the Stanislas Library in Nancy – photography by Clotilde Verdenal ©LoeiLCreatif
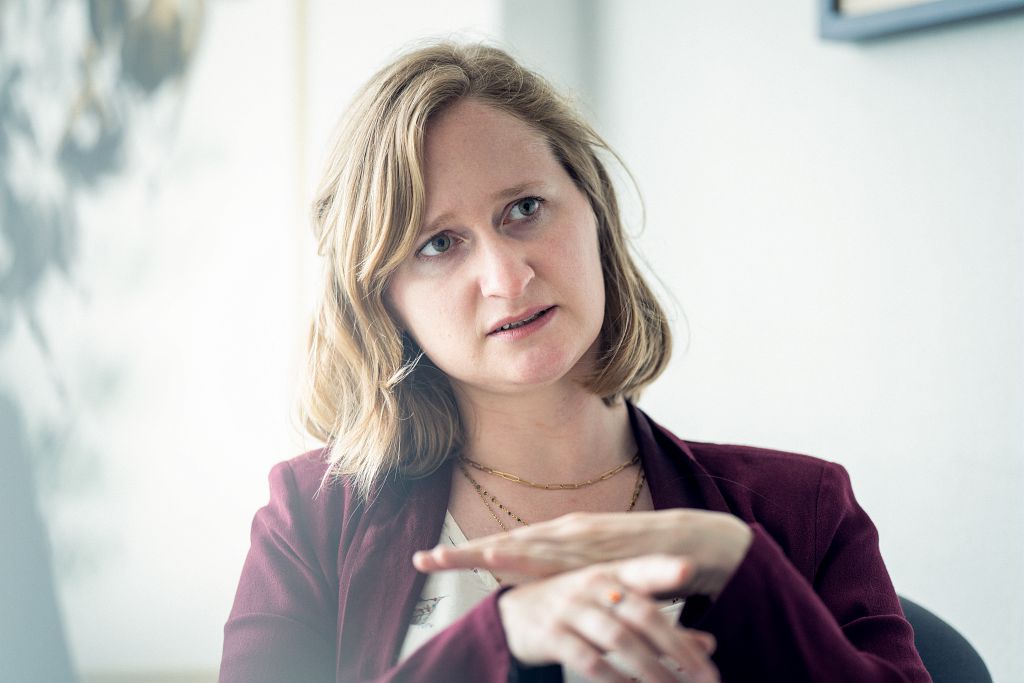
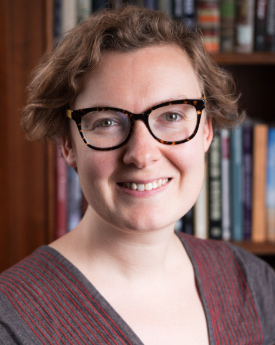