• The new chip, which uses ferroelectric field effect transistor (FeFET) cells, requires 44 times less energy than traditional designs to perform an equivalent number of operations.
• Chips of this kind may later be adapted for cloud computing, but in the near term the main areas of application for the new architecture are in edge computing for transport, industry and IoT.
The large amounts of power required by artificial intelligence systems are a major source of concern for the microelectronics industry, which is eager to develop new chip architectures that are more energy efficient. One researcher who has taken up this challenge is Thomas Kämpfe, a group manager for neuromorphic systems and a departmental manager for components and systems at the Center Nanoelectronic Technologies at Fraunhofer IPMS : “Today, there is a huge demand for Nvidia chips to train and run language models like GPT and diffusion models that generate images and video. And while GPU software architecture has many advantages, companies sometimes need to invest millions of dollars in energy costs to train and run these AIs.” At the same time, industry will have to manufacture dedicated AI chips that compute ever faster and generate less and less heat if it is to extend the scope of artificial intelligence in real-time applications.
An innovative chip architecture that can run on 44 times less energy than the graphics processors used for AI.
Much more modest energy needs
Last year, in an article in Nature Communication published in collaboration with scientists from, among others, Robert Bosch GmbH, the Technical University of Munich, and the Indian Institute of Technology in Kanpur, Kämpfe unveiled an innovative chip design that makes use of ferroelectric field effect transistors (FeFET) that can store information even when they are disconnected from a power source. The new chip also has the key advantage of being able to simultaneously store and process data in transistors, which greatly reduces the bottleneck between data processing and memory.
“The chip we developed with Bosch and Fraunhofer IMPS, which is currently in production in the USA at GlobalFoundries, can deliver 885 TOPS/W”, explains the researcher. For an idea of what this means in practical terms, consider that GPU chips currently used in AI deliver 10 to 20 TOPS/W [Tera Operations Per Second and per Watt]. For the time being, however, the developers of the new architecture are not aiming to replace GPU-based systems but to target a range of uses in edge computing, where AI is deployed at the point where data is collected: in IoT devices, sensors and autonomous vehicles. “One use case, for example, is in automobile systems that pre-analyse objects captured by cameras” without relaying data to a central processing unit. The new chips will therefore open up opportunities to implement AI in highly miniaturized low-latency systems. “In the future, we will get around to integrating them into larger systems,” points out Thomas Kämpfe.
Similarity to the human brain
The design proposed by the research team functions in a manner that is similar to the human brain where neurons process signals and synapses store information. As such, it forms part of a new generation of neuromorphic chips, which feature a variety of biologically inspired architectures for low-power autonomous solutions, among them field programmable gate arrays for oscillatory and impulsive neural networks. Chip designs based on FeFET transistors can now be produced in industrial quantities, however the technology will still need more time to mature. Researchers believe that three to five more years will be necessary before the new chip can be sold on the open market. Much of this time will be devoted to evaluating its safety performance, an important criterion for electronics to be used in drones and self-driving cars. Other less critical applications are also under consideration: “There are other use cases where the lifespan of neuromorphic chips could make a big difference, for example in networks of forest sensors that are required collect data over very long periods.”
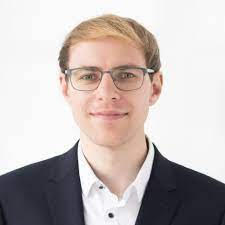