• Christened OpenSafe Fusion, the tool is an automated data fusion framework that combines information from multiple public sources for enhanced sensing of road conditions. This study was supported by the National Science Foundation and the National Academies of Science, Engineering and Medicine’s Gulf Research Program.
• The goal of this research is to leverage enhanced knowledge of conditions on road networks and speed up intervention by municipal authorities and emergency services to protect populations in danger.
In the catastrophic flooding caused by Hurricane Harvey in 2017, more than a third of the victims in Houston died in road-related incidents, which are the leading cause of flood fatalities (40%-60%) in many developed countries. When record-breaking quantities of rain poured down on the Texas city, “there was no real-time information on the impact of flooding on roads,” explains Pranavesh Panakkal of the American non-profit Water Institute, which specialises in applied research on environmental issues linked to water. The researcher is building tools to provide reliable and timely information on the state of urban roadways that will better enable emergency services to organise their relief efforts. This work is based on an AI framework developed in the course of Panakkal’s doctoral and post-doctoral research under Dr Jamie Ellen Padgett at Rice University, which makes use of multi-modal AI inputs. “It is called OpenSafe Fusion which is an abbreviation for Open-Source Situational Awareness Framework for Mobility using Data Fusion. The idea behind the framework is to leverage existing reporting mechanisms and public data sources to predict and keep track of rapidly changing road conditions during urban flooding.”
We included safeguards to enable human operators to check on the model and correct any errors before making use of the data
Nothing like a black box
“Instead of deploying sensors across the city’s road network, which would be prohibitively expensive, the system makes use of readily available data from a variety of sources: CCTV, sensors located on rivers, road cameras that record traffic speed, and posts shared on social networks.” For example, Houston is equipped with a computer-vision AI model that analyses data from 700 traffic-monitoring cameras every five to ten minutes. “Other natural language processing (NLP) models monitor information in public posts on social networks. We analyse a total of nine different data sources, but not all them require processing with AI,” points out the researcher. “Drawing on several data sources increases the accuracy of predictions. At the same time, we didn’t want to build a system that works like a black box, so we included safeguards to enable human operators to check the model’s results and correct any errors before making use of the data.” Results from computer vision models are not always reliable in low light conditions, which is why the approach adopted allows for the adjustment of parameters: “In those cases, we prioritize other data sources.”
A crowdsourced model
Crowdsourced information, which it uses in a variety of ways, plays a key role in the OpenSafe Fusion model:
- it provides a valuable data source in urban areas,
- it facilitates communication between users (for example, among active first responders in the field) and, last but not least,
- it enables stakeholders like the police and emergency services to correct predictions generated by the model if they prove to be inaccurate.
“Stakeholders like hospitals, police, and fire services, etc. need to be able to feed data into the model about flooding they see on the ground. We are also planning to provide a control panel or API, for example for hospitals that need to know more about particular road conditions. The idea is for a high-performance tool that shows zones with reduced access to hospitals, so that emergency services can prioritize intervention in those areas, for example to ensure that outpatients can still be brought in for dialysis.”
Picture: Pranavesh Panakkal and Dr. Jamie Ellen Padgett explaining how their OpenSafe Fusion model works.
Sources :
Pranavesh Panakkal, Jamie Ellen Padgett, More eyes on the road: Sensing flooded roads by fusing real-time observations from public data sources, Reliability Engineering & System Safety, Volume 251, 2024, 110368, ISSN 0951-8320, https://doi.org/10.1016/j.ress.2024.110368
This study was supported by the National Science Foundation (1951821, 2227467), and the National Academies of Science, Engineering and Medicine’s Gulf Research Program (2000013194).
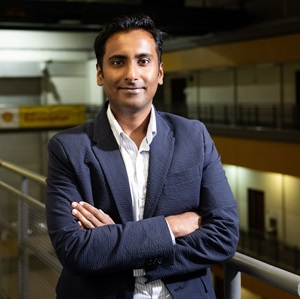