• Researchers projected synthetic 3D anomalies in X-rays to train the model to distinguish between normal cargo and contraband with an impressive accuracy of score of 98%.
• The innovative approach adopted by the project could also be adapted for applications in other fields, including microscopy, medical research and industrial quality control.
Smuggling of illicit goods is a major challenge for many countries, such as the United States, which need to take preventive action to stem the flow of contraband hidden in shipping containers. And with millions of containers moving by land, sea and air each year, it is a daunting task. Bipin Gaikwad and a team of researchers at Tufts University (USA) have developed an innovative computer vision solution to expedite part of this work: “Detecting illegal objects in legitimate shipments is a complex anomaly detection problem. Our AI solution, which complements existing security approaches, does not automate everything,” explains Gaikwad. “However, it does speed up the detection of anomalies relative to expected cargo, enabling X-ray image inspections to be processed more quickly.”
The model was able to differentiate between normal images and images containing synthetic anomalies, based on their visual characteristics
A self-supervised learning framework trained on synthetic data
The AI to detect visual anomalies in container X-rays was developed using a self-supervised learning framework, which reduces the need for the time-consuming and costly process of manually annotating data. To train the model, the researchers artificially embedded 3D anomalies into imagery of normal cargo: “The synthetic dataset met a crucial challenge in self-supervised learning. Rather than using manual annotations, we created synthetic anomalies and projected them in X-rays.” This approach enabled the model to differentiate between normal images and images containing synthetic anomalies, based on their visual characteristics. As a first step, the researchers took datasets of images of grouped items and guided the deep learning AI to identify those that were expected, such as tyres and wine bottles, and those that were not. Initially, the simulated anomalies had relatively simple cylinder and ninja star shapes. Once these were detected, the researchers introduced more complex projections resembling coin purses, animal tusks and jugs. Thereafter: “Our model was able to detect and locate new anomalies that it hadn’t encountered during training, which is essential for applications where anomalies don’t always follow predefined patterns.”
Technological potential in healthcare and quality control
With its key use of residual images, the model has proved to be impressively robust, accurately spotting items that should not be present 98% of the time when tested on several datasets both real and synthetic. And the technology has significant potential beyond the realm of cargo processing. “This framework is versatile and can be adapted to a variety of other tasks in medical research and industrial control,” explains the researcher. With a host of possible future uses, notably the identification of abnormal cells and tissues in MRI and CT scans in healthcare, automated microscopy in medical research, and computerized quality control in industry, Bipin Gaikwad’s research not only marks a major advance in the detection and localisation of contraband that will improve safety and reduce the need for intensive human intervention in transportation systems, but by combining artificial intelligence and self-supervised learning, it opens up promising prospects in a wide range of different fields that involve the detection of anomalies in visual data.
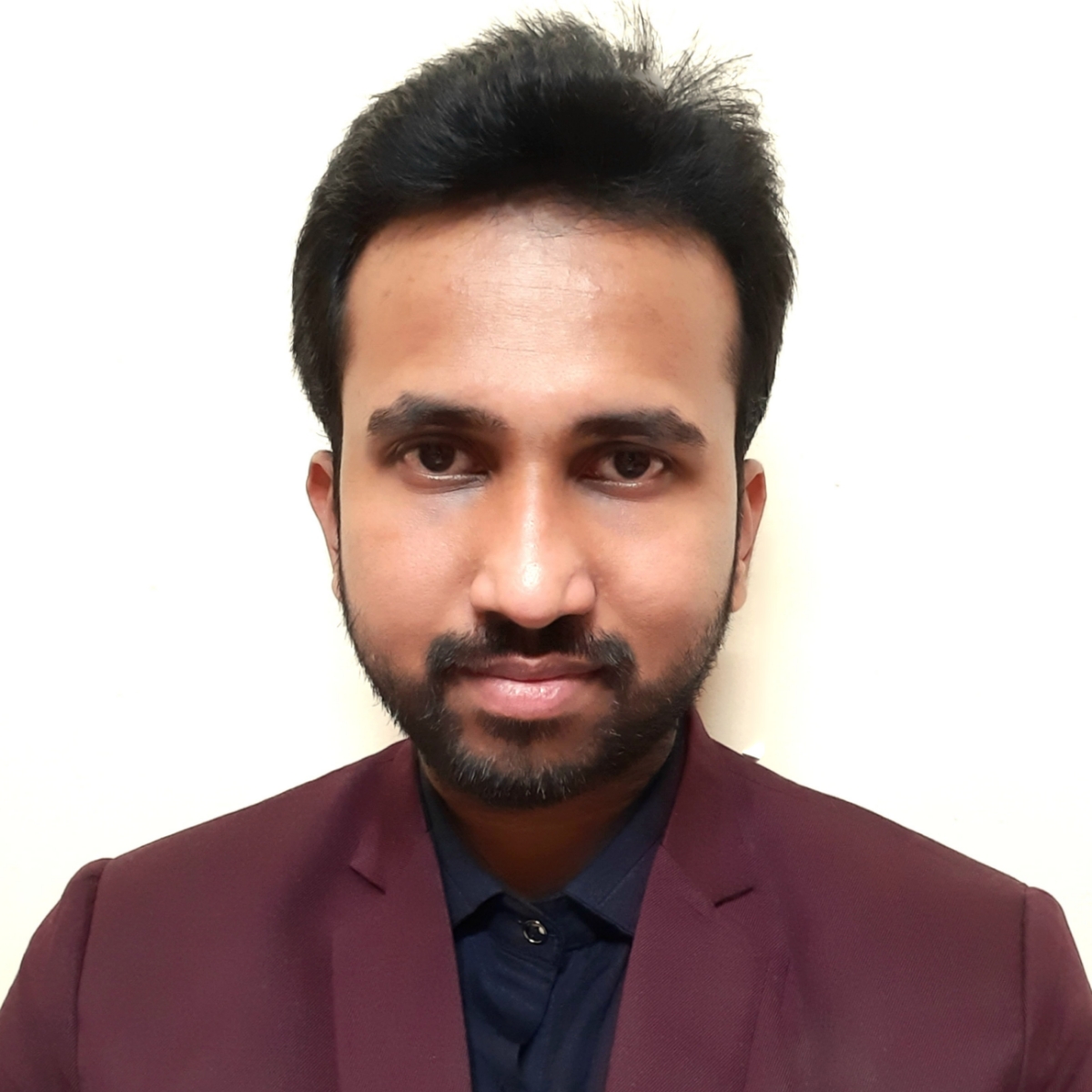