● French start-up Keyprod has developed an IoT device that once attached to machine can provide operators with extensive information on the number of items produced and the condition of parts that may need to be replaced.
● The new tool, which has the potential to boost productivity by between ten and 15 percent, highlights one of the many emerging uses for AI in industry, which is also set to benefit from innovation in computer vision that facilitates the quality control of products before they leave the shop floor.
Understanding how things go wrong at industrial production sites is often an impossible task. Unless you are in a futuristic factory with endless networked sensors, it is difficult to identify causes of malfunctions, which could, nonetheless, have major consequences in terms of safety and productivity, and on the useful life of production machines. “Most of the world’s workshops don’t have any dedicated tools to monitor the operating status of machines, which is done using manual readings,” explains Damien Marc, CEO of JPB Système, an industrial producer of self-locking devices. “One day, I had the idea of monitoring a machine in our factory using the accelerometer on my iPhone, and I was able to collect quite a lot of data. So we decided to create an IoT device capable of interpreting data on vibration, which is the common language of all machines.” And in fact, it is not unusual for technicians to listen attentively to noises made by production tools to understand which tasks they are performing correctly.
The algorithm can independently determine if a machine is functioning correctly, count the number of pieces it has produced, and take note of temporary stoppages that may have an impact on its work rate, etc.
A hardware solution to analyse noise made by machines
Keyprod, a subsidiary of JPB Système, is marketing a hardware solution that combines acoustic and AI technologies to enable users to monitor production effectiveness. These plug and play IoT devices, which can be magnetically attached to any machine, have the capacity to provide predictive data on plant output and scheduling trends. “They are trained to listen to processes and to detect infinitesimal variations in sound, which may result from wear in production tools”: an innovation that has been made possible by artificial intelligence analysis of machine vibrations that can be configured via a control interface. As standard, the algorithm deployed can automatically detect if a machine is running, the number of parts it has produced, and any pauses in production caused by intermittent stoppages, etc.
“We realized that when micro-stoppages are taken into account, machine operation is never fully optimized. The statistics we collect enable us to measure the overall equipment effectiveness for the plant, to see which machines are often stopped, and to work on the reasons why this is the case. At the end of the day, this usually allows us to obtain a gain in productivity of between ten and 15 percent,” points out Damien Marc. “Machine operators can also tag more precise sounds to train the algorithm to recognize them. We can generally obtain a robust model that allows for the precise identification of problems and their causes after two years of using the system.” At its heart, each of these devices has a field programmable gate array that users can configure to perform specific computing tasks. “The advantage of this is that linked devices are programmable and adapted for use with neural networks, which in turn makes them suitable for use with AI.”
AI breaks new ground in industry
The growing use of AI is set to become a major a major trend in industry. According to a study conducted by Augury, 63% of manufacturers are planning to ramp up investment in AI technologies. Companies like Instrumental are leading the way with development of machine-learning tools that can detect problems in the functioning of machines before they become apparent. AI use is also increasingly present on assembly lines, notably to replace human supervision required to detect faults with computer vision. Generative artificial intelligence also has the potential, for example, to help industrial producers automatically identify links between failed products and issues with their design. However, in most cases, and notably with regard to predictive maintenance, human supervision still remains critically necessary.
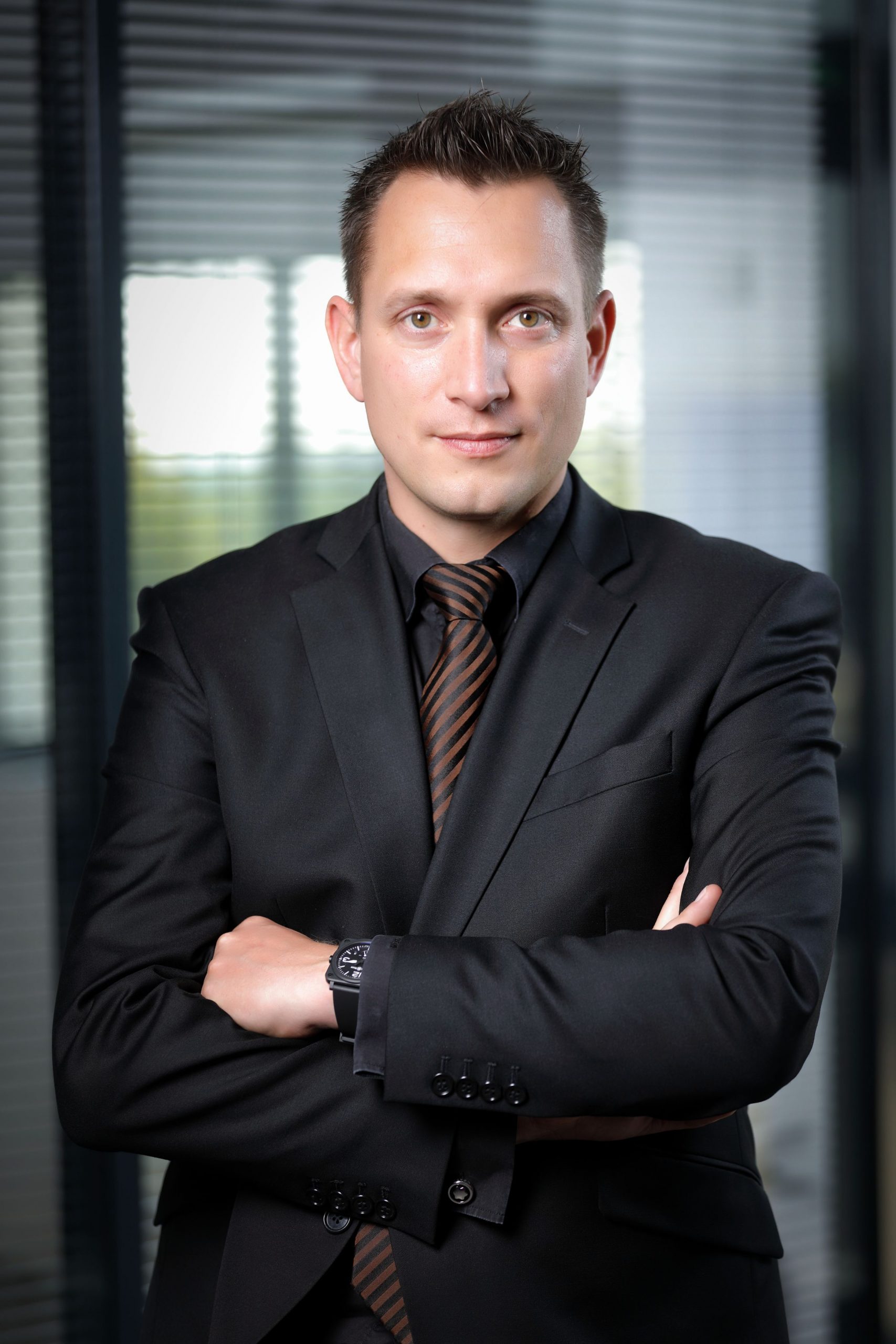