• New research has highlighted the importance of enhancing current detection systems, which are far from infallible, with continuous learning and multi-modal artificial intelligence.
• Some progress has been made on the development of detectors, but extensive research will be required to make these tools, which are typically tested under laboratory conditions, function reliably under real conditions.
“Deepfakes have rapidly emerged as a serious threat to society due to their ease of creation and dissemination,” report researchers from CSIRO, Australia’s national science agency, and South Korea’s Sungkyunkwan University (SKKU). The team has presented a comparative study of deepfake detection tools in an article entitled “SoK: Systematization and Benchmarking of Deepfake Detectors in a Unified Framework”. The conceptual framework they identify focuses on a five-step pipeline for the development of detection tools which it classifies on the basis of the type of deepfake targeted, the detection methodology, the preparation of input data, the manner in which models are trained and model validation. The study also lists18 factors affecting detection efficacy extending from initial data processing to the manner in which detection models are trained and teste. In conclusion, it highlights major vulnerabilities in deepfake detectors under real-world conditions.
Multimodal models integrating audio, visual, and metadata cues could enhance detection accuracy and robustness.
Promising multi-modal models with continuous learning
Specifically, the researchers point out that current training datasets for detectors may leave them “vulnerable to performance degradation against unseen deepfake variants,” to the point where they deliver results that are no better than random guesses. However, their article also indicates: “Multimodal models integrating audio, visual, and metadata cues could enhance detection accuracy and robustness.” As it stands, some deepfake detectors succeed in identifying certain types of counterfeit media but fail to flag others. Not surprisingly, inferior video quality (light levels, noise, resolution) may make detection more difficult. As a general rule, detectors are trialled in labs rather than under real conditions, that is to say they are not tested on “new” deepfakes circulating on the Internet, hence the importance of “continual learning techniques that could enable them to remain effective against dynamic threats posed by deepfakes.” To address these issues the research team has announced that it is developing detection models that integrate audio, text, images, and metadata.
“Current research and innovation has shown that synthetic content needs to be analysed with combinations of different technologies that offer a range of different options for the identification of fakes, and not just a single tool,” points out Vivien Mura, Global CTO for Orange Cyberdefense. A specialist in the field, Mura further explains that these tools are deployed in a scoring framework: “They award a score to content that tends towards real or fake and thereafter it has to be verified by human experts, whose intervention will likely remain indispensable in the future given that a lot of synthetic content is fully legitimate.” Technologies that are specifically developed for the analysis of content can be deployed in tandem with traditional authentication techniques that check user account information and media file metadata indicating codecs and timestamps, etc. “Data like this can help in assessing the reliability of content.”
Alternatives for the identification of deepfakes
“Right now,” points out Vivien Mura, “fake content can still be detected by searching for : , temporal inconsistencies, irregularities in lighting, and mismatches in sound and lighting. Today’s generative models are not yet perfect, particularly in real time.”
Current legislation is strict on identity theft, “however, it does not yet provide robust provisions that impose the obligatory use of in genAI content so that it is easy to detect.” For the moment, detection systems are based on machine learning. “There are generative adversarial networks (GANs) that work, but there is a critical lack of data for video detection, which explains why it is easier to spot fabricated videos of celebrities than deepfakes of ordinary people.” In Mura’s expert opinion, as the volume of artificially generated online content increases and the general reliability of online content decreases, training models to detect deepfakes will likely be even more difficult in the future.
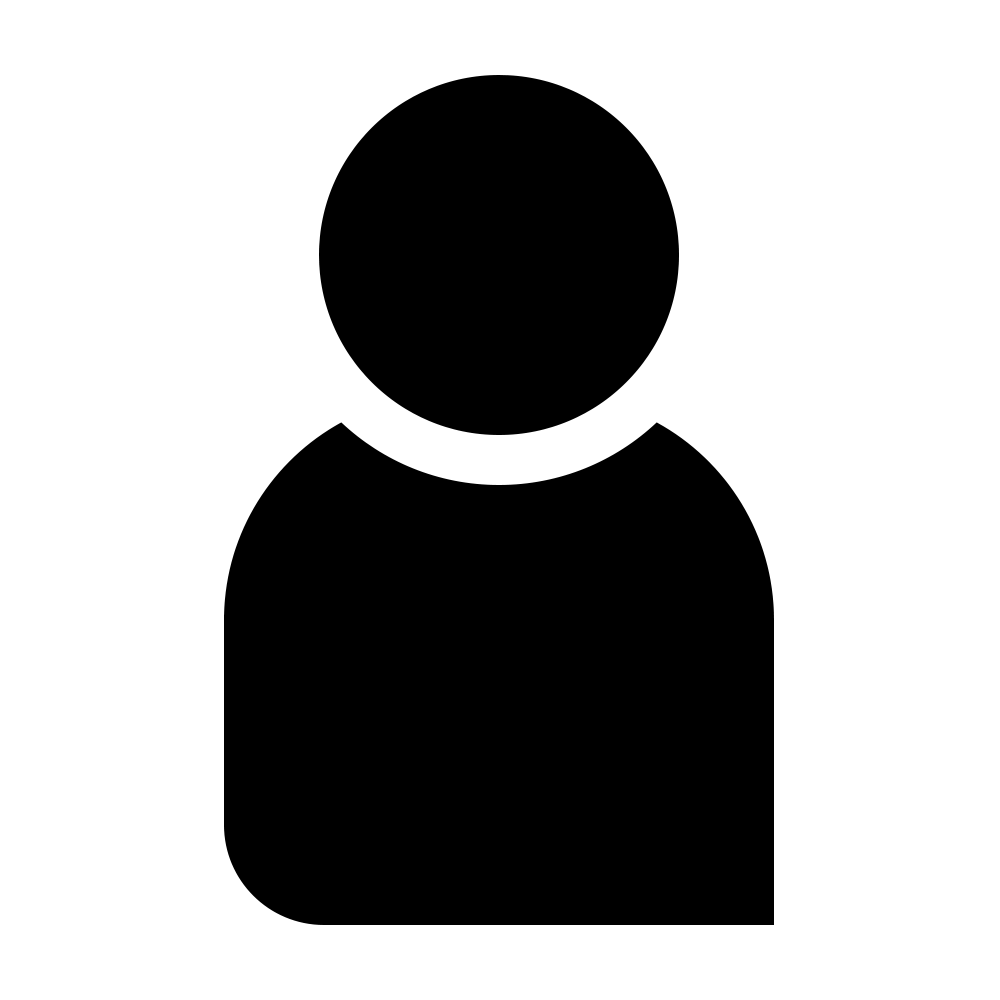